This article is Part 4 in a 5 part series on the fundamentals of DrugOps. In case you'd like to jump directly any of the other sections please find the links below:
Quickly identify issues in antibody drug discovery with Rapid Feedback
A recently-published paper describes an exciting new T-cell engager (TCE) that shows a great deal of promise for treating epithelial ovarian cancer (EOC). Bispecific antibodies are an increasingly popular format for cancer immunotherapy; this paper from last year describes several in development for acute lymphoblastic leukemias and non-Hodgkin's lymphomas, and this Nature article details the substantial deal-making around bispecifics for immuno-oncology. However, adverse events from cytokine release syndrome and on-target, off-tumor toxicity pose significant risks to developing successful treatments.
One of my favorite parts of the paper is the series of failures that informed their design. As the authors describe, folate receptor alpha (FRα) is expressed on a limited subpopulation of epithelial cells and frequently overexpressed in cancer, making it a potential target for epithelial cancers like EOC. Unfortunately, earlier attempts at drugging this target haven’t achieved the desired outcomes. A monoclonal antibody and an antibody-drug conjugate showed some success but failed to meet progression-free survival goals in phase 2 and 3 clinical trials. FRα-targeting TCEs that built on those successes showed even greater promise but caused lung damage in non-human primate models despite the relatively low levels of FRα expression in the lungs.
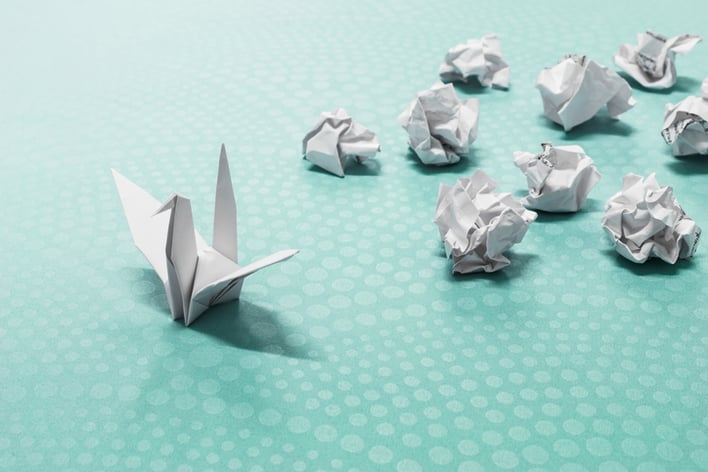
The approach taken for the described TCE was based on development for TCEs against tumors overexpressing CEA and HER2: an avidity-based therapeutic that paired two heavy chains with modest affinity for different epitopes of FRα with a low-affinity CD3 binding arm. This mitigated the on-target, off-tumor risk by ensuring the antibody would only engage when high levels of FRα were present. The low-affinity CD3 arm was able to successfully engage cytotoxic T-cells without inducing the high levels of cytokine release that precipitate cytokine release syndrome.
Of course, failures in drug development are nothing new. What I love is the degree of calibration required to make this particular antibody "just right." Too great an affinity for either CD3 or FRα and tissue damage occurs, too little and nothing happens, all of which must be balanced against potential developability concerns that could surface at any point. The amount of data generated and evaluated, number of avenues considered and potentially abandoned, the engineering efforts directed by the collective experience of developing this particular therapeutic - all of these highlight the essential elements of Rapid Feedback.
Cycles of experimentation occur throughout antibody development. We try different formats, we modify problematic residues, we optimize for different properties. Then we gather data about the changes we made, eliminate the things that didn’t work, and consider additional changes to the things that did. The key to Rapid Feedback is abbreviating this cycle and applying it to everything - including the cycle itself. Instead of waiting for failure, we regularly review data to tell us where we're failing. Then as a team we decide whether that's acceptable. Some projects may not succeed, and some processes may not work. Better to "fail fast" than to continue investing in something destined to become a failure.
In the previous article on Shared Ownership we talked about putting numbers behind our goals and sharing them with everyone, and in the article on Workflow Automation we described automating big chunks of the lead identification process. An ancillary benefit of automation is the ability to gather data that can inform both scientific and operational approaches. The number of clones processed, duplicates removed, and leads identified can tell us what kind of throughput we can generally expect; the per-target prevalence of certain germlines, CDR lengths, or conserved residues can tell us what kinds of antibodies are most likely to succeed against that target.
All of that data then needs to be made actionable - that is, it needs to be sufficient to inform a decision. Understanding the relative enrichment of per-target germlines, CDR lengths, and conserved residues can help us direct our engineering efforts. Understanding our discovery campaign throughput can help us identify process changes we should make. By pairing the data we collect with the goals we set, we can regularly check our progress and inform our decisions about how best to proceed. These are the rapid cycles of iteration and revision at the heart of Rapid Feedback.
Quantitative measures aren't the only inputs. When we talked about Shared Ownership, we concluded with the idea of a retrospective as a form of Rapid Feedback. After addressing obstacles to meeting a team’s objectives, we identify the causes and brainstorm solutions to mitigate future risk without necessarily capturing any specific numbers. Out of these solutions might come a new metric that correlates with an identified cause. This metric then becomes one more piece of data that helps determine whether the company is on track to meet its goals.
Developing Shared Ownership, implementing Workflow Automation, and investing in systems to support Rapid Feedback may sound like a lot of work, but in the same way that DevOps has transformed the way software teams work, we believe DrugOps can do the same for discovery teams. To learn more about how StackWave's affinity software can help support your transition to DrugOps, please watch our Affinity webinar.
To learn more about how StackWave utilizes the fundamentals in DrugOps to shape our approach to laboratory data and workflow management, please check out Part 5 - StackWave Affinity™, A fundamentally different approach to Antibody Discovery.