It has never been more important to organize, standardize, and centralize data collection in the pursuit of new medicines. As a recent article in Genetic Engineering and Biotechnology news explains, "data serves as the foundation of today’s biotechnology and pharmaceutical industries, and that foundation keeps expanding." The sheer amount of data produced during discovery, expression, characterization, and preclinical studies can feel overwhelming, and the disparate tools required to manage all of that data can sometimes impede as much as they assist. Bioregistry software designed to support a team's goals by properly modeling, easily recording, and flexibly querying data is thus an essential component of therapeutic discovery and development.
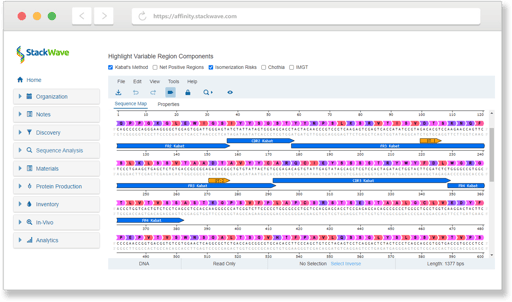
Getting Organized
At StackWave, we've seen many different methods of organizing research data. Some teams begin with one or more spreadsheets. Other teams might have a database expert on hand who can put together a Filemaker or Access database. Still others purchase an electronic laboratory notebook (ELN) and try to shoehorn recordkeeping into it. Let's examine the shortcomings of these three strategies.
First, there's no enforcement of standards. Scientists may use different names for the same thing or different spelling/punctuation for the same name. This leads to duplicate data entry, making it difficult to find information for a project as data for the same therapeutic candidate gets spread across multiple uncorrelated records. The right bioregistry solution forces scientists to use the same terminology, ensures that duplicate molecules are not being recorded, and mitigates the risk of poor-quality data being entered in the first place.
Next, while ELN software may be able to scale as the team grows, spreadsheets and database files do not. Different versions of a spreadsheet can make their way through a teams' inboxes, increasing the risk of information being duplicated or lost. Team members may be forced to spend time reconciling all of these different versions. A file-based database suffers from a similar risk if it's shared in this way, but it also requires either the entire team to learn about database management, or for a single member of the team to be responsible for recording all of the information - typically an untenable approach.
Finally, each of these methods complicates the development of an integrated system for data management and analysis. Many different tools and systems are often required to successfully bring new drugs to market, and the rapid development of AI and ML methods for drug discovery present enormous opportunities for teams positioned to take advantage of them. In each case, the ready availability of properly-formatted data through APIs can be the difference between seamless interoperation and missed opportunity.
Being FAIR
Adopting a bioregistry solution that fits your team and your industry can do more than address the shortcomings identified in the previous section - it can also help make data more FAIR. FAIR data is findable, accessible, interoperable, and reusable. As noted in the 2016 article on this topic in the journal Nature, "Good data management ... is the key conduit leading to knowledge discovery and innovation, and to subsequent data and knowledge integration" by other team members.
By enforcing a single, canonical name for every record and ensuring well-validated input, bioregistries help to make data more findable. Canonical names become the keys that unlock all of the attendant data collected for biological entities in the bioregistry. In addition, a good user interface with simple but flexible search tools make the data highly accessible; such a system simplifies the access of everyone using it, putting more information within reach of more scientists.
Bioregistries with well-designed APIs that can provide data in multiple standard formats are highly interoperable. Other tools can easily obtain the information they need, making it easy to incorporate that information into downstream workflows and analyses. Informatics team members can easily integrate or extend the system through their own scripts and programs.
Finally, if the bioregistry has been designed with the team’s therapeutic format in mind, the data in it becomes highly reusable. A rich and meaningful universe of metadata is available to interpret existing data in new contexts, and connections between biological entities help to develop a "bigger picture" that can drive further insight and action. This data and metadata can also be reused to serve as the foundation for the development or application of AI and ML methods specific to a team’s research.